As we continue our journey in 2024, we can all agree that the world irreversibly changed with the introduction of GenAI. This momentum shows no signs of slowing either, as the mainstreaming of AI continues to advance with an unabated fury. AI can be both potentially transformational and elegantly inaccurate, all at the same time!.
If we take a broad brush stroke, then the rising trends are the ones that accelerate meaningful adoption of AI while the stable trends ensure that we keep improving the underlying data substrate. Declining trends are the ones that do not support the urgency business teams crave to get faster insights from all their data with the least friction and cost.
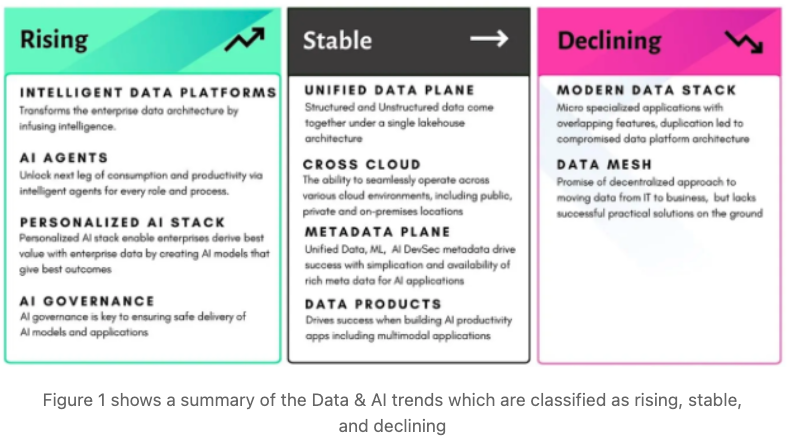
Rising Trends: The rising trends are about plumbing and activation, especially for AI with a focus on data quality, platform architecture, and governance. Autonomous agents and task assistants serving multiple information roles can potentially automate needed activity partly or entirely. In addition, tools for generating high-quality datasets can feed constantly improving models at various stages of the AI model development life-cycle.
- Intelligent Data Platforms: Unifying data warehouses and data lakes to provide a common storage subsystem for structured and unstructured data
- AI Agents: AI-powered programs that can autonomously perform tasks by understanding natural language, reasoning, and taking actions .
- Personalised AI Stack: Customising AI models and responses through fine-tuning, improved datasets, and vector search .
- AI Governance: Ensuring responsible and ethical development and deployment of AI systems
Stable Trends: Increase in data — be it real world or synthetic — puts more pressure on the underlying data infrastructure to continue its strides in becoming more efficient and interoperable. The trends in this section help with simplification of the data and AI stack to make it easier and cheaper to manage and to reduce the burden on organisations from spending an inordinate amount of time on technology and not business imperatives.
- Data Products: Packaging data as a product with clear ownership, quality, and SLAs .
- Converged Metadata Plane: Establishing a common metadata standard to enable data discovery, lineage, and interoperability .
- Unified Data Plane: Integrating batch and real-time data processing for faster, more diverse use cases .
- Cross-Cloud: Running workloads seamlessly across multiple cloud environments for cost optimisation, resilience, and data residency .
Declining Trends: Trends decline as a natural part of the life cycle. They decline when customer preferences or needs change.
- Data Mesh: While the principles are sound, the lack of implementation guidance has led to "data mesh washing" .
- Modern Data Stack: The proliferation of specialised tools has led to a fragmented and high-overhead data stack.
From most of our discussions with our clients, DataGOL emphasises the need to take a hard look at their current data platform architecture and address challenges related to data silos, data quality, duplication, and fragmentation. Building an AI platform, where large language model infrastructure is integrated into the core data platform, will be critical to infusing business intelligence into data applications and accelerating AI adoption
Ready to accelerate your business growth, drive collaboration and up level every decision in your organization through next generation AgentOS? Learn more by exploring the types of Agents you can accomplish through DataGOL, and sign up for DataGOL Platform Trial the Box to see it working. If you are looking for an enterprise ready AI platform and would like to learn more about DataGOL, signup for a personalised demo today!
The DataGOL's Data Intelligence Platform
Unify Data & AI
Democratize insights
Drive down costs
.webp)